Professor Zou, Difan
PhD UCLAAssistant Professor
Tel: (+852) 2219 4614
Fax: (+852) 2559 8447
Email: dzou@cs.hku.hk
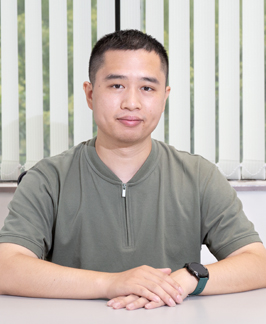
Dr. Difan Zou is an Assistant Professor in the Department of Computer Science at the University of Hong Kong. He received his Ph.D. in Computer Science, University of California, Los Angeles (UCLA). He received a B. S degree in Applied Physics, from School of Gifted Young, USTC and a M. S degree in Electrical Engineering from USTC. He has published multiple papers on top-tier machine learning conferences including ICML, NeurIPS, ICLR, COLT, etc. He is a recipient of Bloomberg Data Science Ph.D. fellowship. His research interests are broadly in machine learning, optimization, and learning structured data (e.g., time-series or graph data), with a focus on theoretical understanding of the optimization and generalization in deep learning problems.
Research Interests
Machine Learning Theory; Deep Learning Theory; Decision Making; Stochastic Modeling; Learning Structured Data
Selected Publications
- Jingfeng Wu*, Difan Zou*, Vladimir Braverman, Quanquan Gu, Sham M. Kakade, Last Iterate Risk Bounds of SGD with Decaying Stepsize for Overparameterized Linear Regression. Proceedings of the 39th International Conference on Machine Learning. (2022) [Long Presentation]
Difan Zou*, Jingfeng Wu*, Vladimir Braverman, Quanquan Gu, Dean P. Foster, Sham M. Kakade. The Benefit of Implicit Regularization from SGD in Least Square Problems. Conference on Advances in Neural Information Processing Systems. (2021) - Difan Zou*, Jingfeng Wu*, Vladimir Braverman, Quanquan Gu, Sham M. Kakade. Benign Overfitting of Constant-Stepsize SGD for Linear Regression. Annual Conference on Learning Theory. (2021)
- Difan Zou, Pan Xu, Quanquan Gu. Faster Convergence of Stochastic Gradient Langevin Dynamics for Non-Log-Concave Sampling. International Conference on Uncertainty in Artificial Intelligence. (2021)
- Difan Zou, Quanquan Gu. On the Convergence of Hamiltonian Monte Carlo with Stochastic Gradients. International Conference on Machine Learning. (2021)
- Difan Zou*, Spencer Frei*, Quanquan Gu. Provable Robustness of Adversarial Training for Learning Halfspaces with Noise. International Conference on Machine Learning. (2021)
- Zixiang Chen*, Yuan Cao*, Difan Zou* and Quanquan Gu. How Much Over-parameterization Is Sufficient to Learn Deep ReLU Networks? International Conference on Learning Representations. (2021)
- Jingfeng Wu, Difan Zou, Vladimir Braverman and Quanquan Gu. Direction Matters: On the Implicit Regularization Effect of Stochastic Gradient Descent with Moderate Learning Rate. International Conference on Learning Representations. (2021)
- Difan Zou, Philip M. Long, Quanquan Gu. On the Global Convergence of Training Deep Linear ResNets. International Conference on Learning Representations. (2020)
- Yisen Wang*, Difan Zou*, Jinfeng Yi, James Bailey, Xingjun Ma and Quanquan Gu. Improving Adversarial Robustness Requires Revisiting Misclassified Examples. International Conference on Learning Representations. (2020)
- Difan Zou*, Yuan Cao*, Dongruo Zhou, Quanquan Gu. Gradient Descent Optimizes Over-parameterized Deep ReLU Networks. Springer Machine Learning Journal. (2020)
- Difan Zou, Quanquan Gu. An Improved Analysis of Training Over-parameterized Deep Neural Networks. Conference on Advances in Neural Information Processing Systems. (2019)
- Difan Zou*, Ziniu Hu*, Yewen Wang, Song Jiang, Yizhou Sun, Quanquan Gu. Layer-Dependent Importance Sampling for Training Deep and Large Graph Convolutional Networks. Conference on Advances in Neural Information Processing Systems. (2019)
- Difan Zou, Pan Xu, Quanquan Gu. Stochastic Gradient Hamiltonian Monte Carlo Methods with Recursive Variance Reduction. Conference on Advances in Neural Information Processing Systems. (2019)
- Pan Xu*, Jinghui Chen*, Difan Zou, Quanquan Gu. Global convergence of Langevin dynamics based algorithms for nonconvex optimization. Conference on Advances in Neural Information Processing Systems. (2018). [Spotlight]
- Difan Zou*, Pan Xu*, Quanquan Gu. Stochastic Variance-Reduced Hamilton Monte Carlo Methods. International Conference on Machine Learning. (2018)
Current Research Project
Machine learning methods, especially deep learning, have been extensively applied in many data-driven real-world applications. Despite the fast growth of the empirical study and application of machine learning methods, theoretical understanding and explanations are still far behind. Besides, most of the recent advances were mainly focusing on improving empirical performance, while the explainability and performance guarantees remain largely unexplored. Consequently, these powerful machine learning system may work pretty well on some particular tasks but may be largely downgraded if transferred to a broader class of tasks. To this end, Dr Zou’s research will focus on the theoretical foundation of machine learning methods and the development of explainable machine learning systems.
In particular, his research will focus on three critical objectives of machine learning.
- Optimization and Generalization foundations of deep learning. This research aims to develop a novel theoretical framework for studying the optimization and generalization in deep learning, including the analysis of the optimization trajectory, implicit bias of the optimization algorithms, and bias-variance trade-off.
- New algorithm designs for training deep neural networks (DNNs). This research aims to (1) develop a comprehensive understanding of the existing training heuristics in deep learning tasks (e.g., normalization, learning rate schedule, adaptive gradients, regularization, etc.) and (2) develop more effective and efficient algorithm designs with insightful motivation and rigorous guarantees.
- Explanable machine learning systems for data modeling and decision making. This research aims to develop explainable machine learning systems for various data-driven modeling in the fields of finance, engineering, and healthcare. In this research, the conventional models (e.g., statistical modeling, physical laws, etc.) will not be entirely discarded but will be effectively incorporated to guide the design of machine learning systems and validate the interpretability of the black-box machine learning models.